In the latest episode of the Silver Linings podcast, Stuart McEwan, Staff Product Manager at FreeAgent, shared valuable insights about implementing AI in accounting software. With nearly a decade at the company, McEwan leads initiatives related to practice and integrations, providing a unique perspective on responsible AI implementation.
The vision behind transaction categorisation
FreeAgent identified bank transaction categorisation as a critical area for automation. As McEwan explains: “One of the core jobs to be done with FreeAgent is to file accurate taxes on time.” The foundational step is correctly assigning transactions to appropriate categories.
In 2019, FreeAgent set an ambitious vision: ensuring accounts were “accurate from day one of using the product.” They aimed to address what customers described as “a red wall of unexplained entries” when first importing transactions without automation.
A data-driven approach
FreeAgent’s implementation leveraged “amazing data sets of hundreds and thousands of millions of user categorised transactions” – not just banking data, but information that had been filed on tax returns and verified by users or accountants.
“We approached it as a supervised learning problem,” McEwan notes, “where we take information from historically categorised transactions to learn patterns and then use those patterns across customers that we don’t know about yet.”
Customer-centric development
The team started conservatively with just four categories that showed clear patterns: travel, bank fees, insurance, and accommodation/meals. This cautious approach proved crucial as they gradually expanded capabilities.
McEwan highlights three key learnings about what customers want from AI:
- “They want AI when categorising bank transactions to be a guiding hand” rather than a cheerleader
- They prefer “demonstration of how to do things rather than absolutely doing everything for them”
- They don’t want AI to impede learning, but to recognise their progress
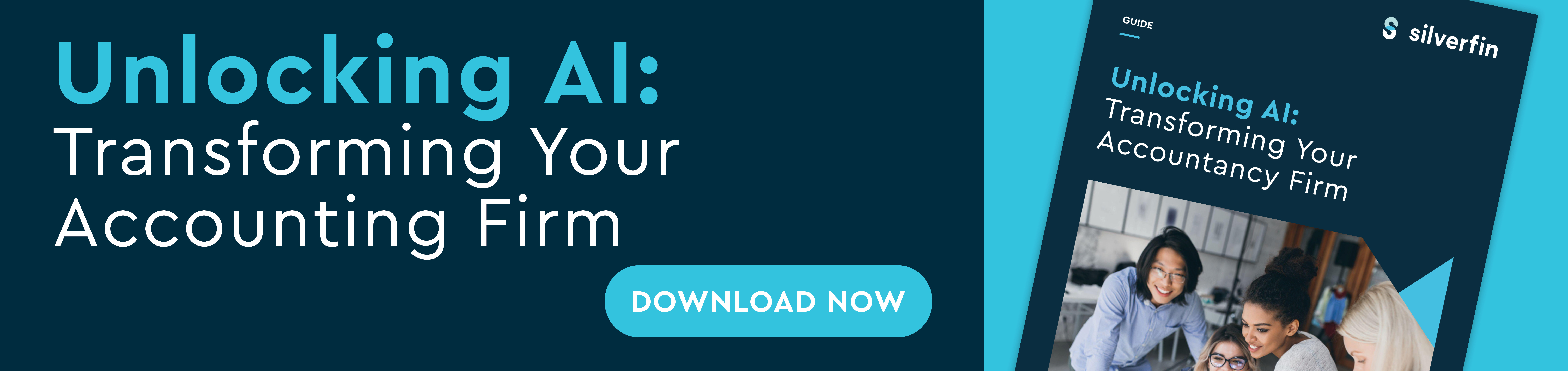
Results and impact
Today, FreeAgent’s AI categorises 65% of all transactions on a user’s first day with about 93% accuracy. McEwan emphasises that they could easily increase this to 100% of transactions, but refuse to do so because “the drop in accuracy, the loss of trust is so important to our users.”
With bank feed adoption at 73% of active accounts, this automation significantly reduces administrative burdens for bookkeepers, accountants, and small business owners.
Trust as foundation
When asked for advice to businesses building AI products, McEwan is unequivocal: “Put trust above everything else.”
He attributes FreeAgent’s success to this principle: “Any time that we thought we would cross the line, and our numbers would be higher in terms of categorisation, but we might lose customers or lose their trust – we didn’t do it.”
As businesses explore opportunities in AI, McEwan’s insights demonstrate how focusing on customer trust and gradual, thoughtful implementation can create solutions that truly enhance user experience rather than simply chasing technical milestones.